Over a period of six weeks near the end of 2021, a multidisciplinary team of KAUST students, researchers and collaborators worked together to solve complex machine learning tasks. The tasks aimed to develop computer codes and algorithms that can sift through enormous amounts of cellular data, filter superfluous information, improve resolution and predict one type of data from another.
The work led to two first-prize awards in one of the world’s most prestigious machine learning conferences: the Conference and Workshop on Neural Information Processing Systems (NeurIPS).
Jesper Tegnér, who supervised the winning team, says “It’s quite remarkable to come from outside the field of biology and to lead the two tasks to the extent that we win the competition.”
“It’s quite remarkable to come from outside the field of biology and to lead the two tasks to the extent that we win the competition.”
Sumeer Khan has a Ph.D. in computer vision and is a postdoc working with Tegnér. Aidyn Ubingazhibov studies mathematics and computer science and was an undergraduate intern at the time. Khan and Ubingazhibov both specialize in machine learning, and their tasks, as part of the NeurIPS competition for December 2021, were to develop algorithms to solve problems in multimodal single-cell data integration.
This is a very recent field of biology developed out of technological advances that allows scientists to measure huge amounts of DNA, RNA and protein data at the single-cell level. Analyses of these data will help explain how our common genetic blueprint gives rise to distinct cell types, organs and organisms, which is key to unlocking disease mechanisms. Scientists hope that the artificial intelligence revolution will expedite these complex analyses.
But developing machine learning approaches for single-cell data is complicated. “When you have this high-resolution data, you also have issues with noise, missing values and imperfect measurements,” explains Tegnér. The huge volumes of available data can compensate for that if scientists can find ways to develop and train machine learning algorithms to filter through the data, normalize it and then analyse it, for example, to classify the cells according to their functions.
KAUST’s winning machine learning models, led by Khan and Ubingazhibov, performed best compared to those submitted by other competitors in two fields.
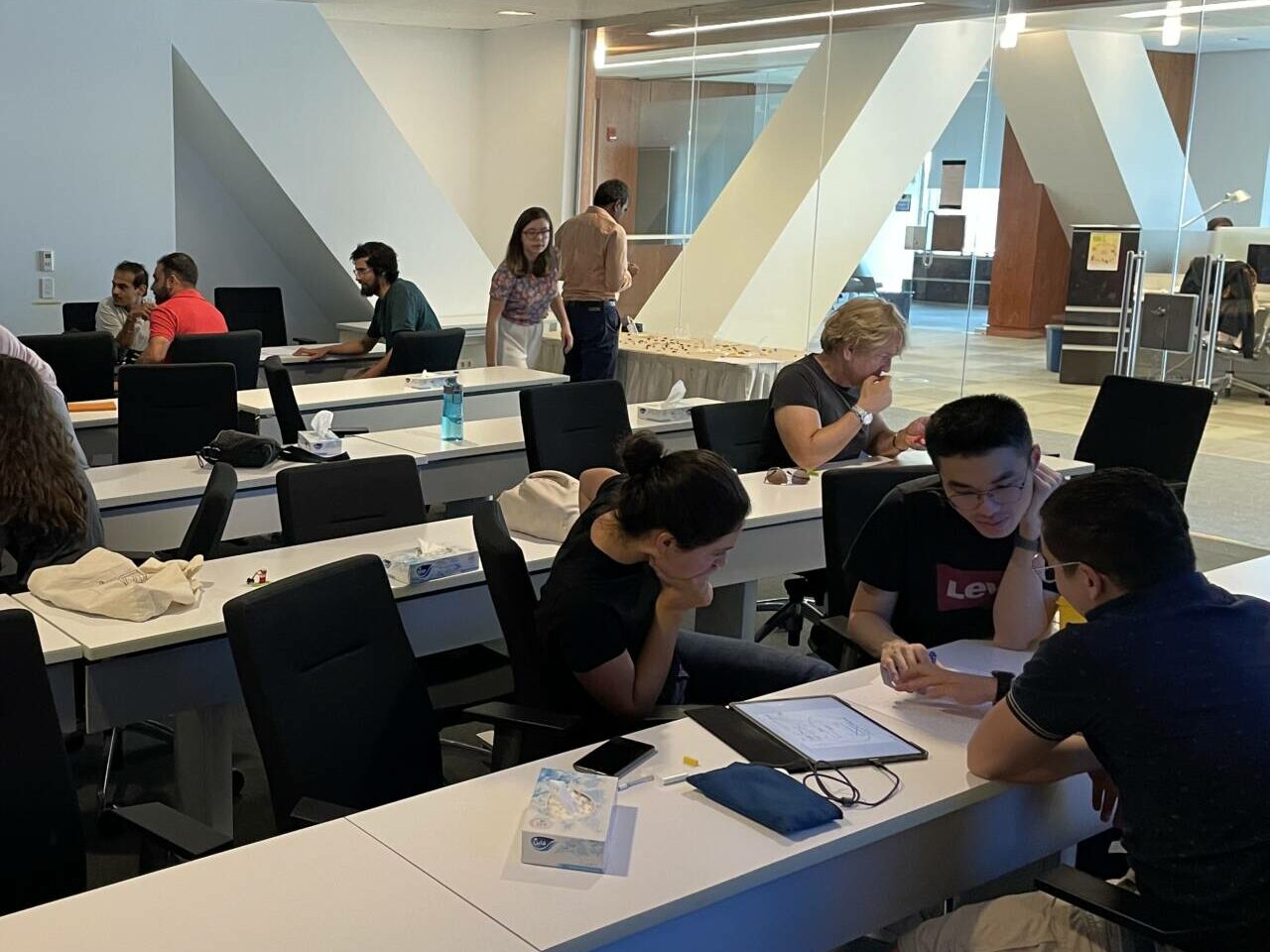
Khan’s machine learning model takes high-dimensional “multi-omic” data related to a cell’s genome, transcriptome and proteome, for example, and learns how to compress it into a low-dimensional “joint embedding” by removing unnecessary noise and cellular batch differences. This sort of method can help scientists integrate different types of data to understand a specific cell’s role in the functioning of a whole tissue.
Meanwhile, Ubingazhibov’s model learned how to use gene expression data in a cell to predict the open chromatin areas in the genome, which determines if genes are accessible to gene-regulatory molecules. Getting better at this sort of task could help scientists develop drugs that can turn genes on or off.
Khan and Ubingazhibov may have led their respective tasks and ultimately submitted the winning machine learning models, but this was definitely a collaborative effort.
“I am a computer science student with no background in biology, so I was a bit nervous at the beginning of the competition,” says Ubingazhibov. “My teammates helped me a lot.”
This help came in the form of regular meetings between a team that comprised researchers with a diverse range of training in biology, mathematics, bioinformatics, genomics, telecommunications, statistics, neuroscience, computer science and single-cell genomics. Algorithms were developed, tested, discussed —and scrapped or improved — until the team was ready to submit their final iterations.
“When you combine discussions about biology and data with this high level of expertise in machine learning and computer science, that’s where you make a difference,” says Tegnér. “That’s easy to say and difficult to do.”
“When you combine discussions about biology and data with this high level of expertise in machine learning and computer science,
that’s where you make a difference.”
Tegnér’s team, co-supervised by David Gomez-Cabrero, associate professor at KAUST, and Narsis Kiani, assistant professor at Karolinska Institute, was building on seven years of experience in integrating multi-omics single-cell data with machine learning techniques. “We have a team that works on these aspects from different scientific angles in a joint sitting on a daily basis. We’re used to this kind of interdisciplinary work.”
Tegnér’s lab combines machine learning techniques with single-cell genomics analyses: to develop new kinds of computational approaches for analyzing and understanding network systems; use the knowledge gained from complex biological systems to create nature-inspired artificial intelligent systems; and disentangle the mechanisms of disease.
“This competition has helped me learn a lot about how to look at the data and tackle a problem,” says Khan. He plans to use what he has learned for his next project, which involves mapping gene expression data across tissues, an emerging field called spatial multiomics. Ubingazhibov agrees that the competition was a great learning process. He next plans to get rigorous training in theoretical machine learning that can be applied in his future journey as a scientist.
Tegnér explains that the knowledge gained from the NeurIPS and similar machine learning competitions goes beyond the personal. “What we learn from this competition is used in our work when we look at our data,” he says. “But also, perhaps more importantly, it defines the most interesting problems to work on and gives the broader field direction for the future.”