“Meeting someone for a relaxed chat at break time often seeds conversations that go beyond the boundaries of departmental meetings or thesis committees. “This is where brilliant ideas are planted!” says KAUST’s expert in high-performance computing David Keyes. One such idea came from geoscientist Matteo Ravasi, who kick started a fruitful collaboration during a coffee break chat with Keyes and colleague Hatem Ltaief.
“My arrival at the university was delayed for a year due to COVID, and so I spent time researching what different KAUST groups were working on. On Github – an online platform that facilitates sharing and co-development of computer code between scientists – I came across recent work by Keyes’ group,” says Ravasi.
A major challenge facing the energy sector is finding efficient, precise ways to image and characterize the subsurface, as well as for uses beyond facilitating oil and gas extraction. Green energy initiatives such as carbon capture and storage and offshore wind farms require accurate knowledge of the subsurface to ensure they are located in the best possible places.
“The size and complexity of seismic datasets, which contain intricate details of underground structures, mean that imaging the subsurface is very computationally expensive,” says Ravasi. “So industry has been unable to utilize the latest algorithmic developments to their full potential.”
This is where the project comes in.
On Github, Ravasi found a matrix algorithm originally developed by Keyes’ group for computational astronomy. Although he did not fully understand how the code worked, he quickly recognized its potential for similar large datasets that are used in seismic imaging.
“This is the fun thing about maths. It is a universal language that transfers across disciplines,” says Keyes. “The code we developed looks for over-parameterizations – essentially repetitions and redundancies – in large datasets and effectively shrinks them in size, without losing precision.”
“This is the fun thing about maths. It is a universal language that transfers across disciplines.”
The researchers began experimenting with the algorithm on seismic data, with the invaluable support of Ph.D. candidate Yuxi Hong. The original code is now embedded in a larger algorithm designed specifically for seismic processing. The algorithm can directly utilize data in their compressed form, improving efficiency in terms of energy and time while yielding high-quality subsurface products.
“In the past, running algorithms to search for carbon storage locations would use so much electricity that it could feasibly generate more pollution than the CO2 we were trying to put in the ground. This computational technique is a real step forward,” says Ravasi.
“We depend on experts who can order data in the best way for compression. We don’t have a deep understanding of cause-and-effect problems in seismic data, for instance, and so we would always collaborate to develop such algorithms,” notes Keyes, adding that “randomly ordered data would not compress at all.”
Collaboration is key to innovation, note the researchers. “You are twice as effective and twice as competent in a team,” says Ravasi. “The KAUST campus is set up so that you can talk easily to one another; living and working together also helps.”
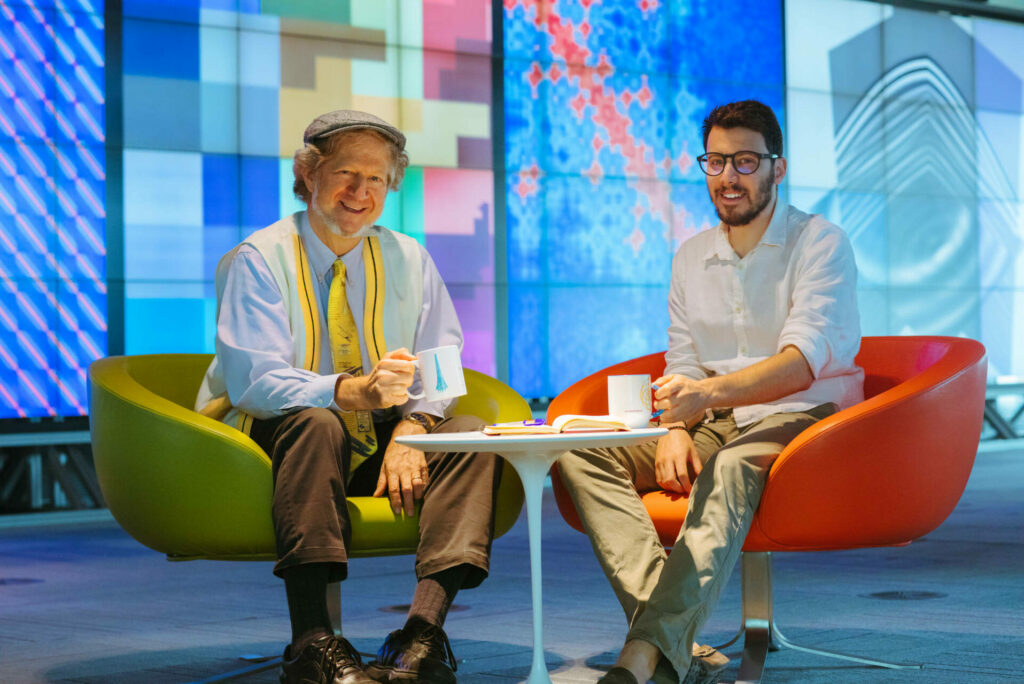
© 2022 KAUST; Anastasia Serin
Collaboration has been at the heart of KAUST since it was founded, points out Keyes. The university is underpinned by a unique management system with a reward structure tied to solving real-world problems, rather than becoming the best in the world in a discipline. As a founding faculty member, Keyes is proud of KAUST’s ongoing commitment to sustainability and its cross-disciplinary missions relating to energy, environment, and food and water security.
There is now considerable interest in the team’s work from the energy industry in Saudi Arabia. “We’re all enjoying riding this particular wave, and hopefully we’ll find more to collaborate on in future,” says Ravasi.